Why Does Data Standardization Matter?
Artificial Intelligence (AI) and Machine Learning (ML) techniques rely on good quality data to optimize their outputs. In a world where these techniques are spreading quickly, scientists still have to spend lots of time cleansing the data they want to consider (removing duplicates, irrelevant data, outliers, flagging missing data, typographical errors) instead of just using it.
Here we highlight an example of high quality, curated data: the Cambridge Structural Database (CSD). This resource is used and trusted by thousands of academic and industrial scientists worldwide. A case study where GSK used proprietary data and the data in the CSD to build ML models is presented, and the importance of data standardization is illustrated.
Sustaining High Quality, Standardized Data
It is well known that X-ray diffraction data are stored in structural databases. The major ones are:
- PDB, Protein Data Bank, with over 200,000 polypeptides, nucleotides and saccharides;
- ICSD, Inorganic Crystal Structures Database, with over 280,000 elements, minerals and metals (no C‒H and C‒C bonds);
- ICDD, International Centre for Diffraction Data, with over 1 million powder diffraction files;
- CSD, Cambridge Structural Database, with over 1.25 million organic and metal organic compounds.
The number of structures in the CSD has been increasing exponentially since its foundation in 1965. The database contains all the experimentally determined published structures of small organic molecules and metal organic compounds, including cocrystals, polymorphs, solvates, hydrates, salts, etc. But what can be learnt from this wealth of data?
By looking at the data in the CSD, valuable insights on the stability and properties of crystalline materials including drugs can be gained, to help mitigate the risks encountered in solid form selection and development [1]. Important questions that can give scientists a greater confidence in progressing a solid form into the development pipeline can be answered, such as:
- Is my structure correct? Is it chemically sensible?
- Is the crystal conformation unusual or strained?
- Is the H-bond geometry distorted? Are the H-bond donors and acceptors accessible?
- Is the H-bond coordination of donors / acceptors optimal? Is there a risk of polymorphism?
- Is the crystal packing efficient? Are there solvent-accessible void spaces?
This structural information can be derived only if the data is in a standardized form, which is what allows the large number of structures in the CSD to be compared and valuable knowledge to be extracted. Curation of the data enables its use at scale, by man and machine.
What is Data Curation?
Data alone, even in a standard format, may not be easily or usefully interpreted by man or machine. Curation adds the context and quality control that allows the data to be easily used and interpreted.
Each entry in the CSD is enriched and annotated by experts at the Cambridge Crystallographic Data Centre (CCDC), who manually check that every structure is chemically correct and make sure that the entries are properly standardized. This validation includes ensuring that:
- The charge in the molecule or compound is balanced;
- Each atom has the correct valence;
- The hydrogen assignment is correct;
- The stoichiometry is correct;
- The units of measurements are standardized (°C vs K);
- The compound name is sensible.
Targeted improvements of the existing entries are also constantly ongoing. As the CSD is almost 60 years old, a manual standardization of the early entries is essential to turn this data into a form that is recognized today, and that matches the information that are currently added to the database.
Additionally, new techniques are emerging in the landscape of crystallography, such as electron diffraction, requiring new field to be added for data standardization. To this regard, it is worth noting that the 1.25 millionth structure in the CSD was the electron diffraction structure of ibuprofen, published in Acta Cryst. A by L. Palatinus et al. (CSD Entry: JEKNOC16).
Underlying data and visual representations have also evolved since the early days of the CSD, and investigations are performed to fix structural diagrams with coincident atoms.
Finally, lots of work is performed aiming to further enrich the entries with more properties such as oxidation state values, crystal habit and colour, and labelling polymorphs in the CSD Drug Subset.
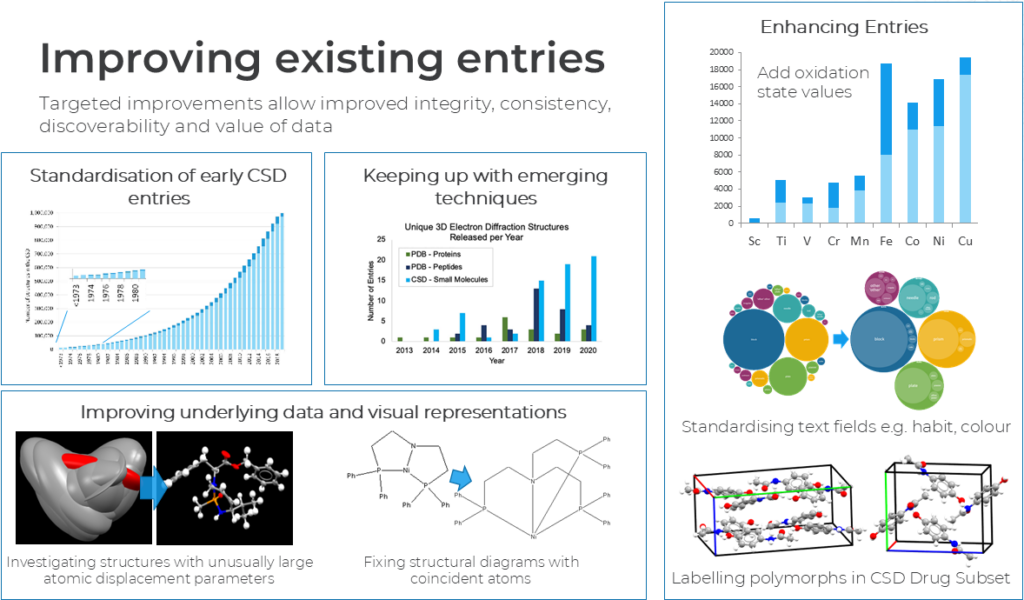
Case Study: Combining Standardized Data from Public and Proprietary Sources
When you have standardized and curated data, it’s easy to combine, search, and unlock real knowledge from it.
In this work reported in CrystEngComm [2], scientists at GlaxoSmithKline (GSK) combined the data from the GSK proprietary database with the CSD Drug Subset to build ML models for the prediction of stable polymorphs and more reliable hydrogen bond propensity (HBP) models.
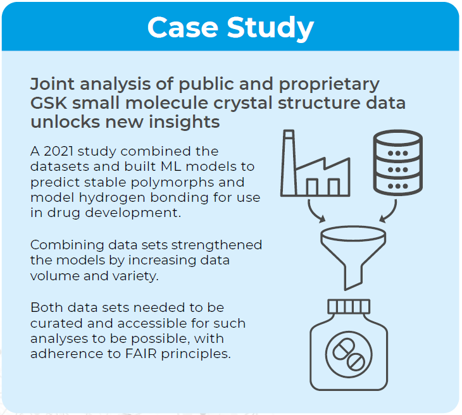
In the work, it was demonstrated that the increased diversity and volume of data within the solid form space derived by the combination of the two datasets strengthened the models. To be able to do that, both the public and private data must be managed to be ‘AI-ready’, and adherence to the FAIR data principles was a key pillar here for data standardization.
The FAIR data principles state that data should be Findable, Accessible, Interoperable, and Reusable: data is assigned a globally unique and persistent identifier; data can be retrieved by their identifier using a standardized protocol; data uses a formal, accessible, shared and broadly applicable language; data is released with a clear and accessible data usage licence. In crystallography, the type of file that is normally used, the CIF (Crystallographic Information File), follows the FAIR data principles, making it data machine and human readable, and fully standardized.
Conclusions
With the increasing popularity and use of AI and ML techniques, having data that is AI-ready has become essential.
Comparing structural properties derived from bad quality data, or data that is not standardized, can lead to incorrect scientific conclusions, loss of trust, and poor business and research decisions.
It is worth investing time and resources in data curation and standardization to derive very accurate predictions and models from the AI algorithms and reach reliable conclusions.
Next Steps
Find out more about the CCDC’s structural informatics tools that access the wealth of data in the CSD to better understand the stability and properties of solid forms. Download our new white paper here: “The Crystal Form Consortium: 15 Years of Industry-led Informatics Innovation”.
Watch this short video “AI and Machine Learning for Chemical Discovery”, which provides an introduction to data for AI and a sample of ML projects using the CSD.
We can help curate your proprietary data! Read more about our data management services, and contact us via this form or email us here.