ML and AI in Life Sciences: Breaking Down Barriers
Here we highlight how Artificial Intelligence (AI) and Machine Learning (ML) are evolving in life sciences, and what leaders in this field can do to support these changes.
The Evolving Reality of AI/ML in Drug Discovery
The pharmaceutical sector strives to deliver safe, effective and profitable medicine to society.
However, the costs to do this keep increasing — despite waves of innovations and investments into R&D. This is due to the high failure rates in the clinical stage, which can reach 90%. How much will AI change this?
Generative AI is nowadays widely adopted in pharmaceutical companies across multiple disciplines, with the most popular investment areas being IT (code generation; code translation) and research (drug discovery; biomarker identification) [1].
However, AI doesn’t exist by itself. It is part of a much bigger change towards a new, digital approach which has long since been implemented here at the CCDC. With a focus on digital transformation — delivering technology solutions to help scientists design better molecules — the CCDC has since its foundation:
- Collated and curated over 1.25 million structural data in the CSD;
- Built predictive tools that leverage this data to create knowledge and insights;
- Supported organizations around the world in introducing their own digital systems to manage their proprietary data.
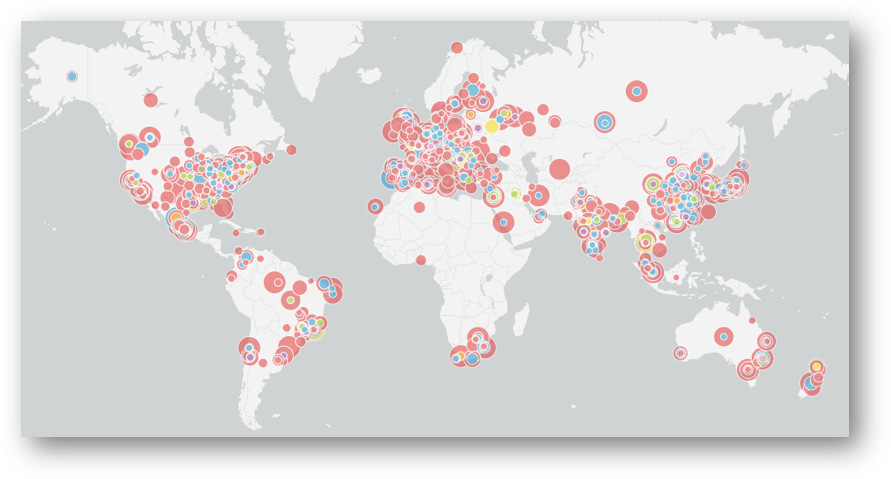
The key to build this “digital first” mindset is to keep in mind the value of change.
Change can often be hard for both an organization and an individual because it means modifying existing behaviours and habits which may have been built up over several years. Despite this, when properly targeted to the strategy, values and purpose of the organization, changing towards a digital approach can help a company achieve its mission faster.
What Can A Leader Do To Support Change?
A leader should clearly communicate a compelling reason that explains why the change is being made, and what value it can bring to the organization.
Leaders should prioritize use cases that align to the overall business goals, defining a clear roadmap for AI to demonstrate how it connects to the strategy of the organization.
Leaders should identify actions that enhance competitiveness, and invest in the future of the organization to keep pace with emerging technologies.
What Are the Enablers?
To make the most of AI, it should be integrated with experimental workflows and other computational tools, and be part of an iterative algorithm-experimentation-data loop that allows us to keep building on our understanding.
High-quality data that follow the FAIR (Findable, Accessible, Interoperable, and Reusable) data principles are the foundation of this loop, as these enable knowledge to be derived and applied across domains.
The right resources, skills, and computational capacity also need to be available, and finally a change of mindset towards a new, digital approach is essential.
“Real transformative change goes beyond episodic efficiency efforts or organizational restructurings. It instead aims to reprogram an organization’s very DNA and embed a new operating model, guided by a bold strategy, to reach a company’s full potential. When done right, a transformation becomes a source of continual renewal, evolving and even revolutionizing a business over a series of horizons.” as stated by McKinsey & Company [2].
Conclusions
AI and ML techniques are evolving rapidly. Managing the change associated with this new digital approach is essential to successfully implement these technologies within any organization.
The focus of this change shouldn’t only be on the AI algorithms, but on the whole organization’s ecosystem that includes infrastructure, systems integration, data, skills, and people.
Next Steps
Watch this short video “AI and Machine Learning for Chemical Discovery”, which provides an introduction to data for AI and a sample of ML projects using the CSD.
To learn more about the 7 factors that must be considered to realize the AI dream in life sciences, download our e-book here.
References
[1] How to Successfully Scale Generative AI in Pharma.
[2] Defining your ‘true north’: A road map to successful transformation.