Improved CSD Torsion Data in GOLD
New Torsion Pattern Library has a Larger and Refined Data Set and is SMARTS Pattern Compatible
GOLD is the validated, configurable protein–ligand docking software for expert drug discovery, used for virtual screening through lead optimization. The torsion distributions sampled by GOLD during protein–ligand docking have been improved, including re-built and enhanced torsion distributions using the latest Cambridge Structural Database (CSD) and Mogul knowledge. SMARTS can be used to define torsion patterns.
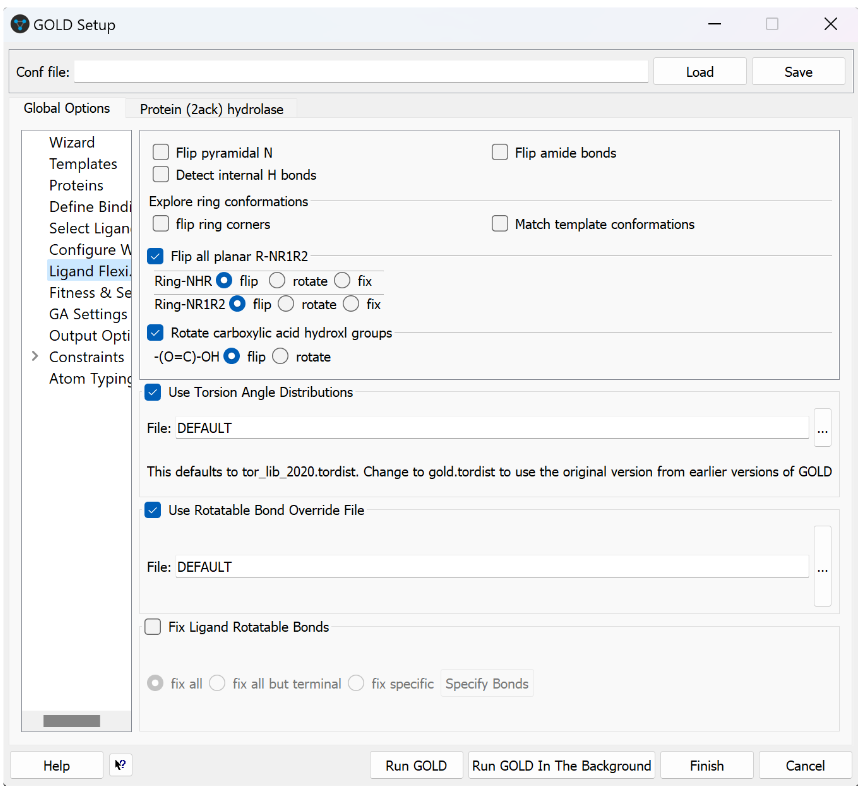

The Benefits
These improvements allow users to find suitable solutions quicker; it reduces the need for post-processing using Mogul (saving time and increasing confidence); it improves the validation results; and the library is SMARTS compatible.
The 1.25M structures in the CSD have been used, generating 439 patterns and giving a library of 389 distributions. They were used to demonstrate a reduction in post-processing where now Mogul only has a fraction of violations after docking. This has also benefited the top-pose ranking in GOLD enabling even higher confidence in the docking outputs.
What is New?
The torsion distributions sampled by GOLD during protein–docking have been improved including re-built and enhanced torsion distributions using latest Cambridge Structural Database (CSD) and Mogul knowledge. Torsion distributions are a collection of torsion motifs associated with angle distributions, derived from crystallographic databases. They are used in strain assessment, conformer generation, and geometry optimization (see J. Chem. Inf. Model. 2022, 62, 7, 1644–1653).
A common task in GOLD protein–ligand docking is to post-process the results with Mogul to filter out poses that Mogul would mark as unusual. Could this be avoided if we were to just use more torsion patterns with more stricter settings?
SMARTS patterns have now been introduced to define torsion angles, along with a script to generate libraries from a list of SMARTS patterns. A large subset of the patterns from the paper by Scharfer et al. along with a further 46 more specific patterns, have generated a library of over 500 distributions used to evaluate this method.
To test if these patterns have any impact on protein–ligand docking, the old default library was evaluated against a set of structures taken from the CSD Drug Subset to see how much coverage the previous default library had. Only 40% of rotatable torsion angles were covered by a distribution in the default library. If the more extensive set of SMARTS patterns was used, the coverage increases; with >98% of rotatable torsion angles covered by one of the patterns in the new library.
Does more coverage of the torsional space make a difference in protein–ligand docking? To test this, a docking experiment was conducted where the CSD Drug Subset was docked into a protein target (PDB entry 5LMA – human tyrosene kinase) to see if the number of Mogul unusual torsions in the results falls. With the old library, it was seen, on average, 0.68 unusual bonds across a set of dockings into a standard protein target.
With the new patterns, similar results were initially obtained because GOLD is highly discerning with torsion data, using an estimate of the relative energy of a torsion profile. This method is somewhat problematic as it over-emphasizes distributions with lower numbers of hits.
Further work was carried out to explore better ways of using the patterns directly. A method that uses the area occupied in the distribution for each bin in a given histogram has been adopted.
Initially, the torsion distributions were generated using the patterns from Scharfer et al. and this had a moderate effect in GOLD. Further investigation suggested that several patterns in that paper were general; by removing the general patterns and adding some more specific patterns (for example, for peptides) significant overall improvements were gained when docking the CSD Drug Subset. With the few extra patterns to the Schafer paper that were more specific, the average unusual bonds per docking dropped from 0.68 to 0.33, i.e. the number of mogul violations halved in the outputs, representing a significant improvement.
The new patterns also impact the recapitulation of the top ranked pose. The performance of the codes were re-evaluated on the cleaned up Astex Diverse set with the previous default library (gold.tordist) and the new codes and library. With the previous default library, using 30% efficiency settings, 63.4% of top-ranked poses were within 2.0 Angstroms RMSD. With the new library this increased to 68.8% for this same test set. (Please note: the numbers should be taken with some caution, as there is some variance from run to run due to the stochastic nature of GOLD). It is possible to force more compliance with Mogul by using higher cut-off parameters when performing docking.
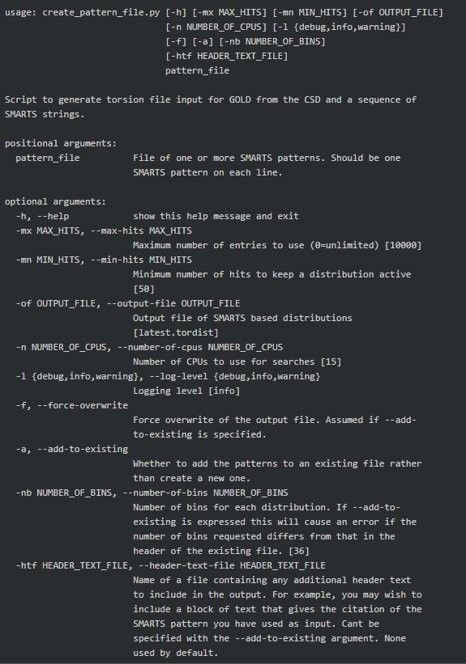
Next Steps
Request a demo of the CSD and/or CCDC software that supports scientific discovery, development, and analysis, and is trusted by thousands across industry and academia.
Read more about GOLD, the validated, configurable protein–ligand docking software for expert drug discovery. For virtual screening through to lead optimization.
Find out more about Mogul, the software that provides a rapid, accurate assessment of molecular conformations in the context of millions of experimental observations.
Learn more about the Cambridge Structural Database (CSD) – the comprehensive repository of validated and curated small molecule organic and metal-organic crystal structures.