CSD in Action: Exploring Protein–Ligand Docking Improvements in Flexible Ring Structures
Successful protein–ligand docking depends upon two factors – the exhaustive exploration of search space, and effective scoring.
In this blog we explore the improvements that can be gained in both pose prediction and scoring using the validated, configurable protein–ligand docking software GOLD.1, 2, 3 The conformational space of flexible ring structures that commonly form the core of small molecule drugs and biological substrates is examined.
One area where the ring template matching methodology is envisaged to be of particular use is in the docking of ligands containing macrocycles.
Method
The ring template matching algorithm in GOLD relies on finding a matching template in the ring template library. The ring template library was derived from the Cambridge Structural Database (CSD). During ligand initialization, each flexible ring was checked against the ring template library. If there was a match in the library the templates for that ring were sampled during the genetic algorithm.
In this study, protein–ligand complexes (PDB IDs 1S19, 2PCP, 1KI8 and 1TT1), where the ligand had a ring capable of adopting different conformers, were obtained using the cavity comparison tool (accessible through the CSD Python API).
Non-native conformations of the ligands can be generated using the conformer generation tool (accessible through Mercury or the CSD Python API).4 The non-native ligands were then docked back into the protein binding site using four different protocols:
- Without either the ring corner flipping or the ring template options turned on
- With the ring corner flipping option turned on
- With the ring template matching option turned on
- With both the ring corner flipping and the ring template matching options turned on.
In order to ensure exhaustive exploration of conformational space each ligand was docked 25 times using 10 genetic algorithm runs, resulting in 250 poses for each ligand.
Results
One example where sampling ring conformational space proved to be crucial for obtaining the correct pose is in calcipotriol binding to the vitamin D3 receptor (PDB ID 1S19). The flexible ring did not form part of the central core of the ligand, but is located at one of the ends of the elongated ligand (Figure 1). The ring conformation generated was not able to form key hydrogen bonds, and as such, the wrong pose was obtained when not sampling ring conformational space. The correct pose was obtained by either using the ring corner flipping or the ring template methodology.
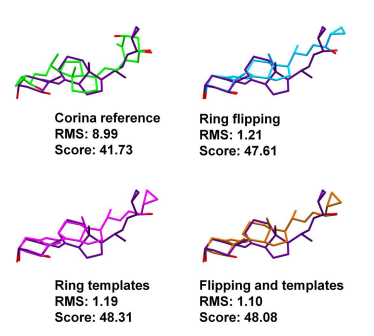
Another example that illustrates how a flexible ring conformation can drive the overall pose is PDB ID 2PCP. A simple inversion of a six membered chair conformation was all that is required to obtain the correct binding mode. The ring corner flipping or the ring template matching algorithms work equally well (Figure 2).
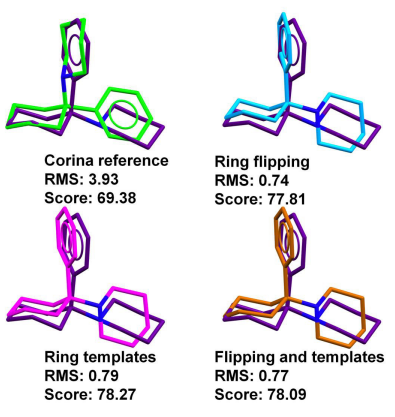
In some cases the value of sampling ring conformational space was not necessarily apparent when using the overall RMS as a measure of success. In the case of PDB ID 1KI8, docked using the PLP scoring function, the ring corner flipping algorithm results in an average fitness score increase of over 7 units. This can be attributed to improved Van der Waals interactions (the shape fitting component of PLP) and the formation of an additional hydrogen bond. This is in contrast to the improvement in the RMS, which is relatively small at 0.47 Å (Figure 3).
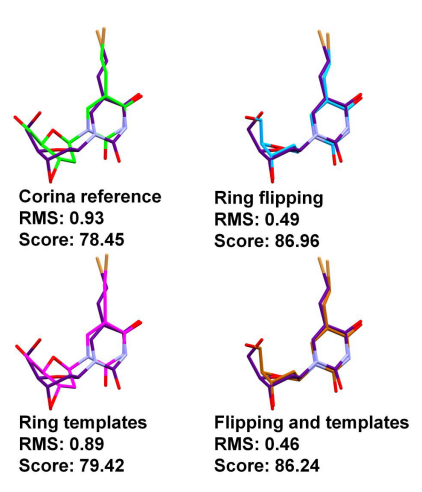
What is also demonstrated by the PDB ID 1KI8 example is the behaviour observed when using both the ring corner flipping and the ring template matching algorithms in conjunction. In the case of 1ki8 the improvement in the fitness score is much lower when using ring template matching than when using the ring corner flipping algorithm. When using the two algorithms in conjunction the appropriate pose is retrieved.
Another example highlighting the dramatic changes that can be achieved in the fitness score by small variations in the binding pose is provided by kainate binding to the glutamate receptor (PDB ID 1TT1). The improvements in GoldScore with respect to the reference are 12.48, 14.58 and 20.50 using the ring corner flipping, the ring template and the combination of the flipping and template methodologies respectively. However, the differences in the RMSs are never greater than 0.45 Å (Figure 4). The improvement in the fitness score, with respect to the reference, is due to the hydrogen bonding term. Interestingly, the combined use of the ring corner flipping and the ring template methodologies does in this instance result in a ring conformation that was impossible to retrieve using either of the two methodologies independently.
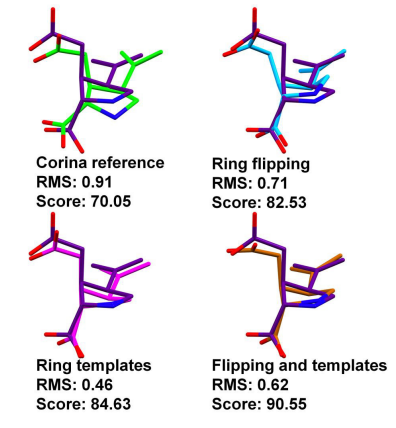
Conclusions
The GOLD ring corner flipping and the ring template matching algorithms provide different approaches to sampling the conformational space of flexible rings. The ring corner flipping algorithm has the advantage of being able to provide a systematic, although limited, search of the ring conformational space. The ring template matching algorithm is to an extent less systematic in that it relies upon pre-existing conformations being available. However, it has the advantage that it can produce small subtle changes in the ring conformation, which may be required to optimize the interactions of protruding functional groups. The two algorithms can be used in conjunction.
Virtual screening experiments are dependent upon the fitness score generated by the docking program. As such the observation that sampling ring conformational space can lead to large improvements in the fitness scores, sometimes whilst the overall RMS does not change much, is of particular importance in virtual screening (Figure 5).
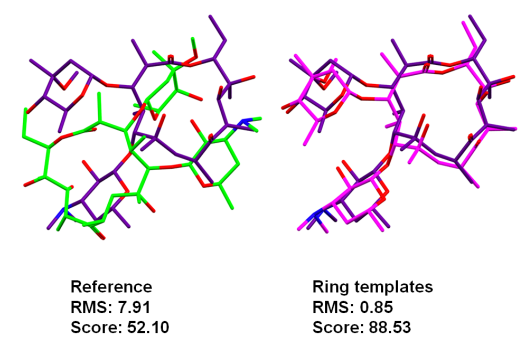
References
- H. Goto and E. Osawa, J. Am. Chem. Soc., 1989, 111, 8950-8951. https://doi.org/10.1021/ja00206a046.
- A.W.R. Payne and R.C. Glen. J. Mol .Graph., 1993, 11, 74-91. https://doi.org/10.1007/BF00124408.
- G. Jones, P. Willett, R. C Glen, A. R Leach, Robin Taylor, Journal of Molecular Biology,
Volume 267, Issue 3, 1997, Pages 727-748, ISSN 0022-2836. https://doi.org/10.1006/jmbi.1996.0897. - J. C. Cole, O. Korb, P. McCabe, M. G. Read, and R. Taylor, Journal of Chemical Information and Modeling, 2018 58 (3), 615-629. https://doi.org/10.1021/acs.jcim.7b00697.
Next Steps
- More information on GOLD, the validated, configurable protein–ligand docking software for expert drug discovery.
- Read another GOLD case study – Next Generation Ovarian Cancer Drug Identified by GOLD Virtual Screening and Confirmed Experimentally.
- Download the GOLD white paper – Ultra-large GOLD Docking on Cloud Resources.
Tags
CSD (154)
Database Group (30)
Drug Development (46)
Drug Discovery (62)
GOLD (19)
Pharmaceuticals (46)
Tools in Action (37)