Modeling Melting Points for Aqueous Solubility Prediction
Today’s blog is based on the webinar “Building Machine Learning Small Molecule Melting Points and Solubility Models Using the CSD Dataset” presented by Dr Valery Polyakov from Meliora Therapeutics.
In the webinar, Dr Polyakov illustrated research results from the collaboration between Sutro Biopharma and the Cambridge Crystallographic Data Centre (CCDC) that was published in May 2023 in Journal of Chemical Information and Modeling.
Preface
Dr Valery Polyakov is an expert in drug discovery and information technology, with a strong focus on leveraging programming languages and tools, as well as molecular modeling and cheminformatics techniques.
With extensive experience in the pharma industry with companies such as Sanofi and Novartis, including late-stage development support, Dr Polyakov specializes in building models for multi-parameter optimization.
Dr Polyakov has published 38 papers in peer-reviewed journals and holds 22 patents/published patent applications.
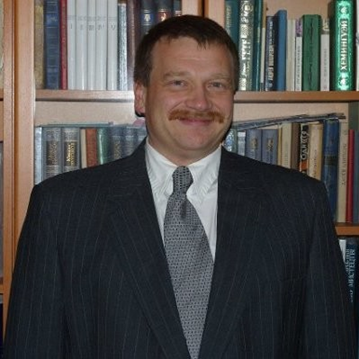
Advantage of Using Melting Points for Solubility Predictions
Aqueous solubility is a key parameter that for the characterization of an Active Pharmaceutical Ingredient (API) during the drug discovery process and beyond.
If a compound is soluble, the assay results and analyses performed are reliable, and no problems will be encountered when formulating the drugs for oral or IV administration.
Poorly aqueous soluble compounds are a major problem encountered in formulation development of a new chemical entity, leading to issues with mechanism of action and bioavailability. There are still a lot of drugs that are poorly soluble (Figure 1).
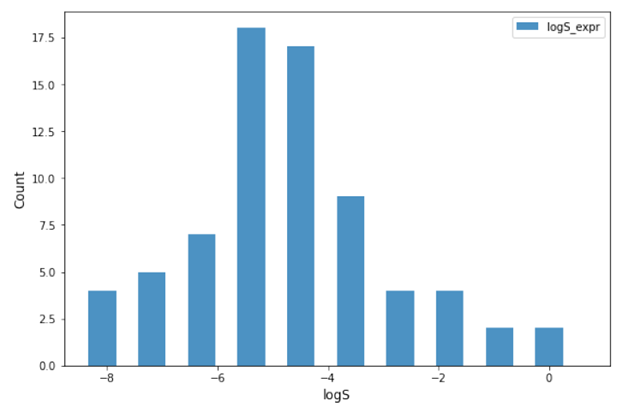
The study of solubility by modeling melting points rather than through the measurement of solubilities has distinct advantages. Firstly, solubility datasets are small and inconsistent. Secondly, measuring solubility is more complex than measuring melting points, as many factors can impact the measurements, such as the sample crystallinity, or polymorphism, leading to high errors between different measurements.
It is important to note that dissolution involves a first stage in which the crystal packing of the crystalline solid is broken, and a second stage in which the solute interacts with water.
How Did the Models Get Developed?
The workflow of the melting point and solubility modeling that was used in the work is reported in Figure 2.
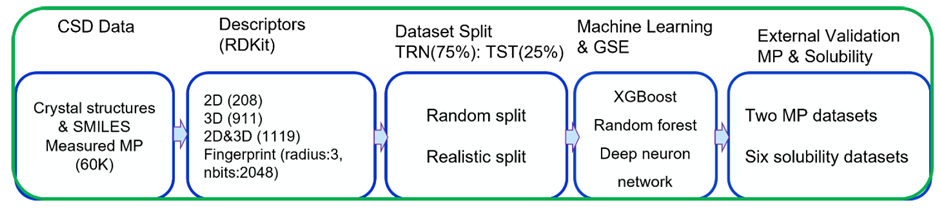
The CCDC provided access to the Cambridge Structural Database (CSD) data containing melting points. In March 2022, when the analysis was conducted, the CSD contained 170 K organic and metal-organic compounds with experimental melting points. As the purpose of the research was to build models for virtual screening of small organic molecules, metal-organic compounds were excluded from the study. Further data polishing was done to remove salts, mixtures, hydrates, molecules with melting points lower than room temperature or higher than 377 °C. This led to the identification of 58,810 unique crystal structures with associated melting points suitable for model development.
As the melting process of a compound involves the breaking of intermolecular forces which are related to aspects such as size and constitution of compounds, 2D, 3D and fingerprints descriptors were then calculated to improve the model performance.
The models were then tested with both random split and realistic split to evaluate their quality. The first consists in splitting the dataset randomly to test the trained set; the second is more sophisticated, and consists in clustering the compounds first, building the models on the large clusters, and finally testing the model in smaller clusters.
The next step of the workflow was the evaluation of the study through three machine learning methods for regression: eXtreme Gradient Boosting (XGBoost), Random Forest (RF), and Deep neural network (DNN).
Among the series of models developed for the melting points, the ones involving XGBoost with 2D descriptors gave the lowest mean absolute error (MAE, average of the difference in module between the experimental value and the predicted one), and hence the best results. The advantage of using 2D descriptors, rather than needing to use the 3D conformation for molecules, allowed the expansion of the dataset to 100 K structure by including mixtures, salts and hydrates.
As it can be seen from Figure 3, the models show high prediction accuracy in the drug-like region, between 50~250 °C, with a minimal overprediction for melting points < 150 °C and underprediction for melting points > 150 °C.
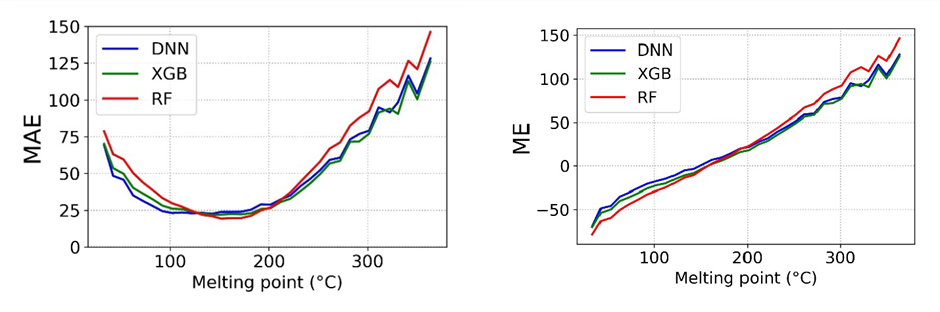
Validation of Melting Point Models and Performance of Solubility Models
A validation of the melting point models using two completely external published datasets was then carried out to confirm the predictive power of the models. Once again XGBoost performed the best for the validation datasets, showing the lowest MAE, while DNN showed the highest MAE.
Six published datasets with solubility data were found for solubility validation, all containing a different number of compounds. In five of the datasets, XGBoost outperformed all the other methods used, which also included tools to predict solubility already reported in the literature (ESOL, a linear model proposed by Delaney widely used to predict solubility based on simple physicochemical properties; and SFI, solubility forecast index).
For the sixth dataset, all the models showed no prediction. PROTAC compounds represents in fact a challenge due to their considerably large molecular weight.
Internal Application of the Solubility Predictions
After completing the workflow of the melting point and solubility modeling, researchers at Sutro Biopharma developed a tool to incorporate melting points and solubility predictions in the company database. The solubility and melting points of compounds started being assessed before registration in the database, and the models were rebuilt for more accurate predictions when additional experimental melting point data were added.
Conclusions
An efficient workflow to develop and assess machine learning models to predict melting points and solubilities was presented. The Cambridge Structural Database (CSD) was used in this work, representing the largest ever dataset investigated for this purpose. Five out of six external datasets validated the effectiveness of solubility predictions obtained with the machine learning models developed. The model failed when investigating molecules with extremely large molecular weight, representing its one limitation.
Next Steps
To discuss further and/or request a demo with one of our scientists, please contact us via this form or .