Leveraging the Structural Data Bridge for Digital Drug Design
This blog discusses how experimental data across repositories are bridged to facilitate cross-database mining, enabling new discoveries and de-risking the drug development process.
Structural Databases
The Cambridge Structural Database (CSD) contains over 1.2M experimental 3D structures with data from X-ray and neutron diffraction analyses. Researchers across the pharmaceutical, agrochemical and fine chemical industries use the database to predict and guide future discoveries. The CSD is a trusted scientific resource that gives big-data insights using powerful algorithms for molecular analysis.
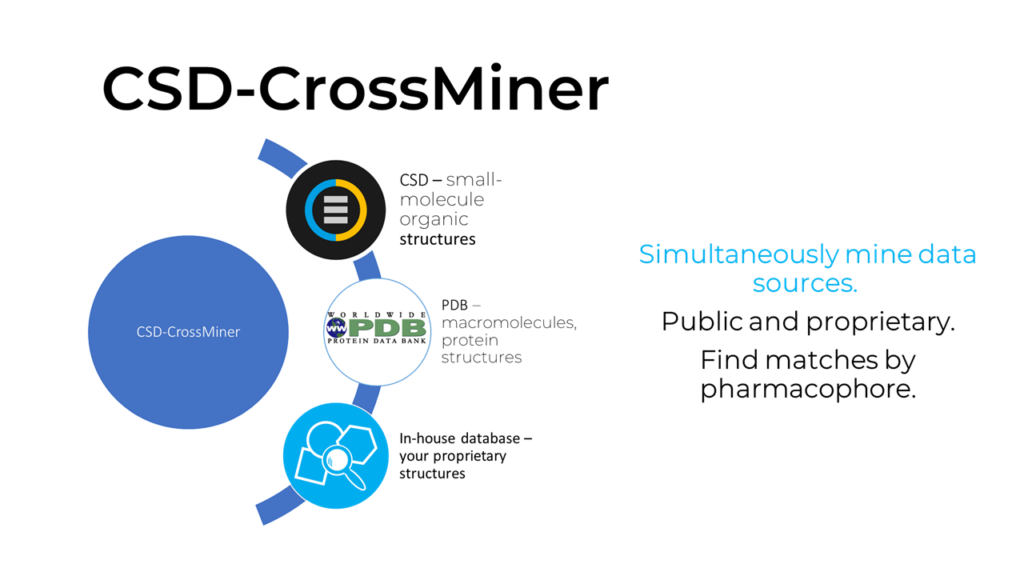
The Protein Data Bank (PDB) is the world’s repository for protein crystal structures. CSD-CrossMiner was developed to close existing gaps between the CSD and the PDB.
To know more about impetus behind CSD-CrossMiner at its inception, read the original research.
Scaffold Hopping and CSD-CrossMiner: The CSD Advantage
Creating the right compounds in the earliest stages of your drug discovery efforts is critical to avoid pitfalls, such as those related to pharmacokinetics (PK), in the later stages of the drug development process. Using advanced approaches, you can optimize based on your needs with minimal expenditure. CSD-CrossMiner is an informatics-based software, drawing on insights from the CSD and PDB experimental data, while not requiring excessive compute power.
CSD-CrossMiner helps you find the “right fit” from the outset of your drug discovery endeavours. It is used in:
- Hit-to-lead screening
- Identifying off-target effects
- Lead optimization
- Understanding modifications that are tolerated in the binding site
- Finding scaffold hops to improve pharmacokinetics (PK)
- Generating new ideas and hypotheses
CSD-CrossMiner leverages a carefully selected subset containing 400,000 drug-like structures from the CSD. These are organic and fully defined, and the subset is free of disordered structures. CSD-CrossMiner further incorporates structural data from the PDB (see Fig 1). It contains extracted protein-ligand binding complexes from the PDB, including protein and the nucleic acid complexes. Mining in-house data is also possible with CSD-CrossMiner through its custom database functionality.
Workflow: how to use CSD-CrossMiner
Using CSD-CrossMiner to build pharmacophore models is straightforward.
First, bring in the protein-ligand complex of interest and define the ligand and protein pharmacophores, as well as any constraints. This is quick to do with pre-defined click-and-place features. Then, query the relevant databases and see matching hits overlaid, which can each be explored in turn (see Fig 2).
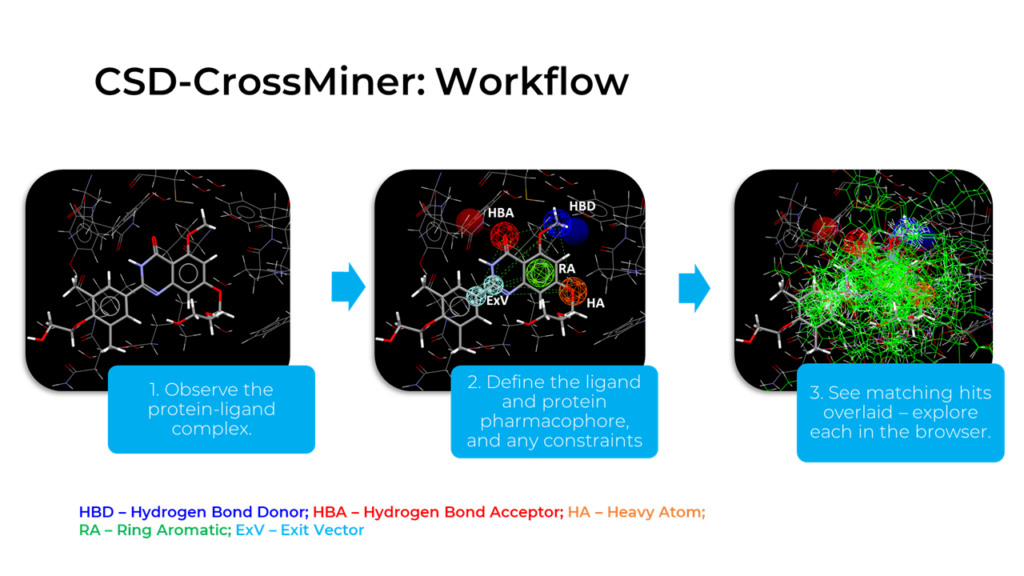
With tailored queries, the potential for exploration is vast. In the following case study, we kept important interactions intact and looked for other functional groups with the potential to improve on a promising drug that failed in phase III clinical trials.
Case Study: Identifying Potential Bromodomain containing Protein 4 (BRD4) bromodomain 1 (BD1) Novel Selective Inhibitors
BRD4 belongs to the family of Bromodomain and Extra-Terminal (BET) family proteins. Epigenetic regulatory proteins play critical roles in the regulation of gene transcription. BRD4 is extensively studied and a promising drug target for anti-inflammatory therapeutics. Potential drug RVX- 208, interacts with a conserved asparagine (Asn140) in the active site, and its water molecule-mediated interaction with tyrosine (Tyr97) is also observed. However, RVX-208 failed in phase III clinical trials. Sub-optimal PK and lack of specificity for bromodomain 1 (BD1) are thought to have played a role in this failure.
Can scaffold hopping with CSD-CrossMiner suggest modifications to improve its performance?
In CSD-CrossMiner, we tailored our query to improve these aspects by focusing on additional interactions identified by Liu et al (see Fig 3). We’ve used the BRD4—RVX-208 crystal structure (PDB ID: 4MR4) as our reference and labeled our residues and water molecules of interest. We then built our query, adding our pharmacophore features and respective tolerance radiuses (see Figure 4).
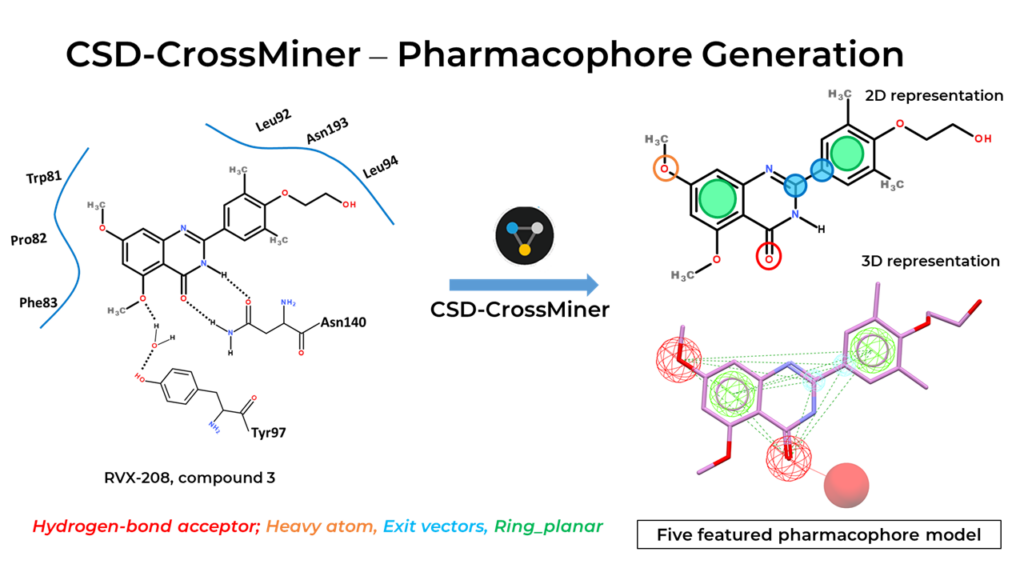
Using CSD-CrossMiner to model what an optimized compound might look like, we were able to replicate the findings published by Liu et al. We also found other scaffolds that could be used in further optimizations for potency and selectivity.
Leveraging CSD-CrossMiner at the earliest stage of your project is a straightforward, low-cost yet powerful means to mining the available structural data for new insights.
Next Steps
Watch the full webinar ‘Scaffold Hopping in Digital Drug Design’ here.