Catalyst Ligand Design using CSD-CrossMiner
Researchers from the University of Leeds and the Cambridge Crystallographic Data Centre (CCDC) have developed a computational workflow for ligand discovery in catalysis with the Cambridge Structural Database (CSD), as reported in Catalysis Science & Technology. In this work, CSD-CrossMiner is used for catalyst design and development, highlighting the power and versatility of the tool that is usually used to identify lead compounds in pharmaceutical development.
Introduction
Catalytic processes involving precious metals such as Pd, Ru and Rh are well established in the literature. On the contrary, the development of base metal catalysts and suitable ligands is still a challenge for the world of organometallic catalysis.
The use of computer-guided search can help with the choice of the optimal ligands for specific catalytic applications. High-throughput experimental approaches are efficient when looking for suitable ligands from libraries of compounds, hence being limited by the available libraries. In silico ligand investigation explores instead the entire chemical space, potentially revealing novel and unexpected ligands.
In this work, the CSD and CCDC software tools are used to explore the ligand space in a relevant catalytic reaction, avoiding the experimental feasibility challenge, while accessing a wide space of chemical compounds.
The Catalytic Reaction
The reaction investigated is the copper(I)-catalysed Ullmann–Goldberg reaction (Figure 1), a C-N cross-coupling that occurs in milder and more sustainable conditions compared to the equivalent palladium-catalysed one. The latter, the Buchwald–Hartwig coupling reaction, is preferred for its reliability and well-developed ligands. Several reaction mechanisms were instead proposed for the copper(I)-catalysed reaction, depending on the substrates and ligands used. This, added to the unexplored role of the ligand in influencing parameters such as the catalyst stability or reaction yield, were further investigated.
The Study
This study involves the use of the CSD to perform automated ligand discovery, and high-throughput calculations to investigate the energy barriers of the catalytic reaction studied. Among the proposed reaction mechanisms, the group chose to study the ones involving the two transition states reported in Figure 1, the oxidative addition (TSOA) and sigma bond metathesis (TSSig). The choice derived from the simpler description of the transition state complexes and more accessible computational costs.
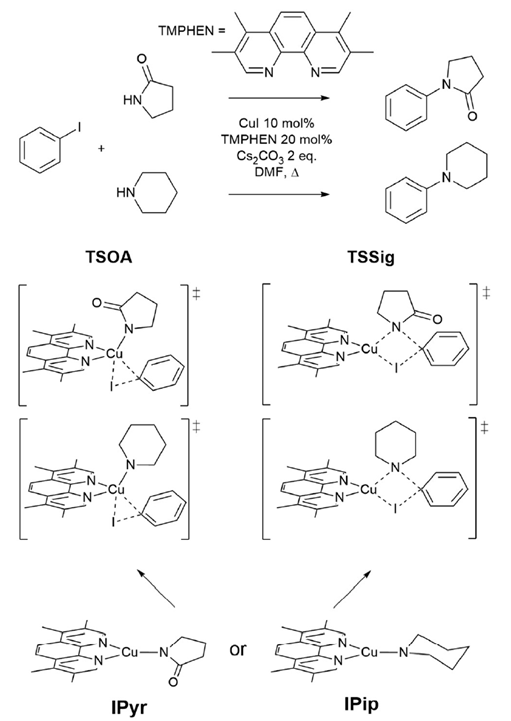
Methodology
The workflow for high-throughput catalyst design starts with the identification and curation of the literature ligands. The Reaxys database allowed the extraction of the ligands involved in the Ullmann–Goldberg coupling reactions. After identifying approximately 20 000 reactions, data polishing was performed by removing ligands contained in precatalysts and removing the reactions with no yields or identifiable ligands (from compounds such an nanoparticles). Finally, 345 unique ligands were identified, with structures retrieved as SMILES.
The ligands identification from the CSD was performed with the CSD-CrossMiner tool. A 3D structural query called catalophore was carried out using feature points to describe the transition state of the ligand (Figure 2). This search was performed using some new features of CrossMiner written by the authors of the work, specifically targeted to organometallic structures. Further polishing and filter application, such as the removal of polymers and structures with disorder, was applied to the structures obtained, leading to > 32000 potential ligands.
The CSD Python API was used to automate the process. The catalytic intermediates IPyr and IPip, and the transition state TSOA and TSSig were generated, and their structures optimised through the Python toolkit, allowing the generation of the transition state templates.
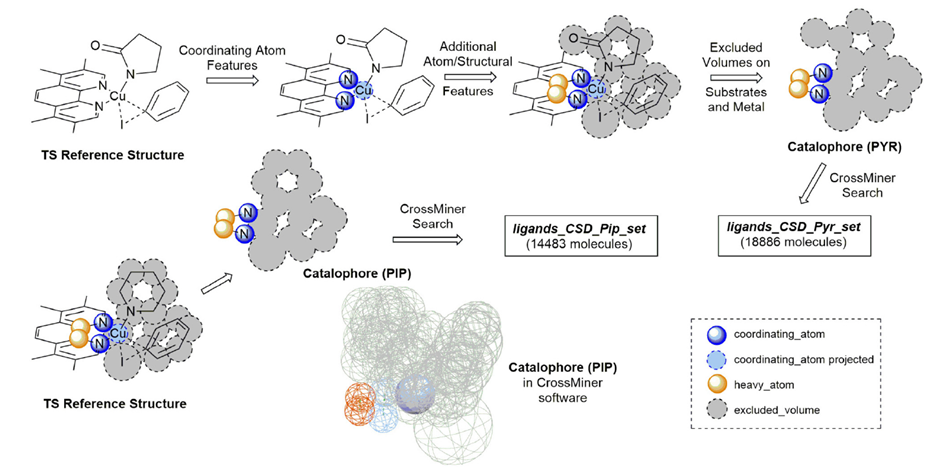
Choice of Computational Method
The use of high-throughput computational methods to study organometallic complexes and transition states is not extensively reported in the literature. For this reason, scientists decided to use a variety of semi-empirical and DFT methods to investigate the geometries of the Cu(I)-based compounds.
The assessment of the quality for the optimised structures was performed by considering the metal-ligand bond lengths and ligand-metal-ligand bond angles. More specifically, the parameter considered was the mean absolute error (MAE) between the experimental and the calculated values, meaning that a low MAE represents a more accurate model. Additionally, the computational costs were considered as well, identifying the GFN2-xTB and B97-3c and the most suitable computational methods for the study of the Ullmann–Goldberg reaction.
The ΔG‡ for Cu(I)-based catalysts constructed with the ligands extracted from the CSD were then calculated. The B97-3c method revealed to be the best for balance between computational time and accuracy in the calculation of activation energies for the studied catalytic reactions. The following step was hence the generation of the catalytic intermediate transition states for each of the ligands, and the validation of the computed transition states.
The application of the workflow to the entire set of Cu(I) compounds, allowed to identify ligands that are not suitable for the Ullmann–Goldberg coupling reaction. For example, in the case of finding and optimising TSOA as transition state, the success rate was quite low (33%), which was in agreement with what reported in the experimental literature. TSSig is in fact less sterically demanding than TSOA, leading to a better success rate.
Computational Descriptors and Machine Learning
A wide range of computational descriptors for machine learning (ML) were chosen to describe steric and electronic properties of the investigated compounds and transition states.
Eight ML algorithms were then employed to the datasets, allowing to identify the extra trees (ET) algorithm as the one which gave the best metrics, followed by random forest (RF), bagging (Bag) and support vector machine (SVM) giving comparable results.
The generation of ML models allowed to predict the ΔG‡ values accurately, with 70.6–81.5% of predictions included within ± 4 kcal mol−1 of the calculated ΔG‡. The addition of descriptors derived from TPSS/def2-TZVP//GFN2-xTB calculations further improved the accuracy of ML models to 75.4–87.8%, providing an excellent balance between accuracy and computational time.
Conclusions
A new high-throughput computational workflow for ligand/catalyst development was presented.
The workflow investigated Cu(I)-catalysed C–N coupling reactions, and allowed to generate organometallic transition states, perform computational calculations to determine structural and electronic properties, and to predict ΔG‡ for the investigated compounds.
This workflow outperforms existing methods for its speed and accuracy, and represents hence a method that can be widely applied across multiple chemical areas of catalysis design.
Next Steps
To discuss further and/or request a demo of CSD-CrossMiner with one of our scientists, please contact us via this form or .
Read the full article here: Catal. Sci. Technol., 2023,13, 2407-2420.