Artificial Intelligence (AI): Facilitating Solid-State Drug Formulation Development
This blog is based on the article ‘Emerging Artificial Intelligence (AI) Technologies Used in the Development of Solid Dosage Forms’ published in Pharmaceutics in 2022.
Introduction
Artificial Intelligence (AI) is a versatile tool that simulates human intelligence using multiple algorithms, and hence using computers. The concept was introduced in 1956 by Marvin Minsky and John McCarthy, and has since been widely applied in the pharmaceutical industry to facilitate some of the challenges that can be encountered during the formulation development process.
Active pharmaceutical ingredients (APIs) are most commonly formulated into solid-state forms such as tablets, capsules, powder, and granules. The development of solid dosage forms is complex, containing several processes in which numerous factors like solubility, polymorphs, stability, excipient compatibility, dissolution and scale-up must be considered. AI represents an efficient approach that can be used to optimize the pharmaceutical formulation development process, representing a powerful strategy when compared to the conventional trial-and-error pathways. Furthermore, pharmaceutical industries have invested in AI companies aiming to develop better drugs and medical devices (Figure 1), proving that the use of AI has already improved the decision-making process, alongside research and clinical trial efficiency. Nowadays, several AI-based products have also been approved by the FDA, who published an action plan in 2021 focused on the regulatory oversight and on improving the life of patients.
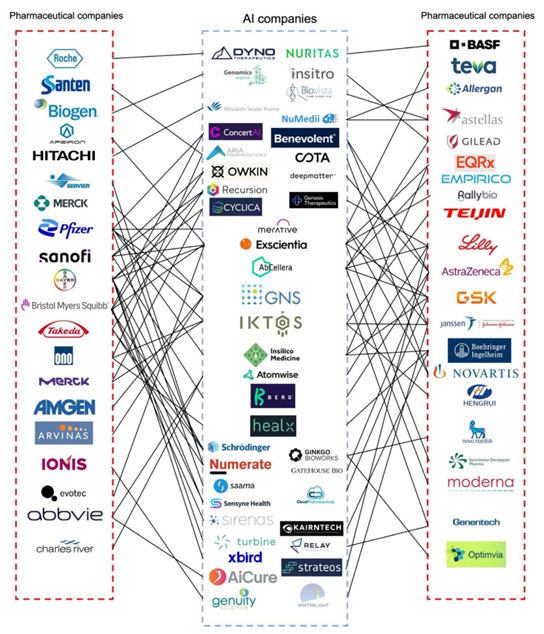
Commonly Used Databases and Data Processing Methods
The starting point to perform an AI-based analysis is the creation of a high-quality database, which is necessary to develop a suitable model for formulation development. As can be seen in Table 1, the Cambridge Structural Database (CSD) is one of the open-source databases that contains information on solid dosage formulation, alongside PubChem, one of the most widely used website for chemical information, and Drugs@FDA, which contains drugs and biological products that are approved in the U.S.
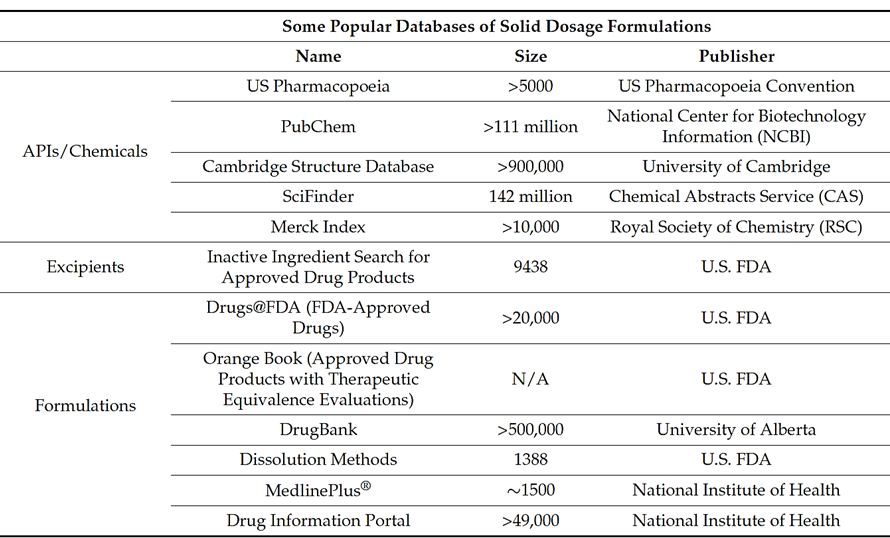
Once the database is created, the data is processed via methods that allow to adjust them before being used. Examples of these methods are data cleaning, used for missing or inaccurate dataset observations; dimension reduction, used to remove features that are less important to reduce the complexity of the model; imbalanced data solutions, that are applied when the distribution of different classes within the database is unequal; and data splitting, that consists in randomizing the dataset and dividing it into subsets.
Finally, the data must be converted into machine-readable formats. The International Chemical Identifier (InChI), the Simplified Molecular-Input Line-Entry System (SMILES), and the Molfile (MDL) are three of the most important molecular representation methods. These allow chemical identities to be encoded according to their chemical composition and atomic configurations and have been incorporated in the AI algorithm to reliably generate molecular representations.
AI Algorithms in Solid Dosage Forms Development
In recent years, a variety of algorithms have been used for pharmaceutical solid dosage form development. The algorithms are divided into subclasses according to their type of classification within the machine learning (ML) group, a subfield of AI. ML can be classified into supervised learning, which contains the regression and classification algorithms; unsupervised learning, containing clustering and dimension reduction algorithms; and reinforcement learning, which comprises decision making algorithms. Finally, deep learning (DL) represents an additional subfield of ML including state-of-the-art algorithms such as artificial neural networks.
Each algorithm presents advantages and disadvantages, and it is important to identify the optimal ML algorithm for the modelling process once the data processing step is done. Table 2 represents a summary of the characteristics of each algorithm that can guide to the selection of the suitable algorithm as a starting point for the model development.
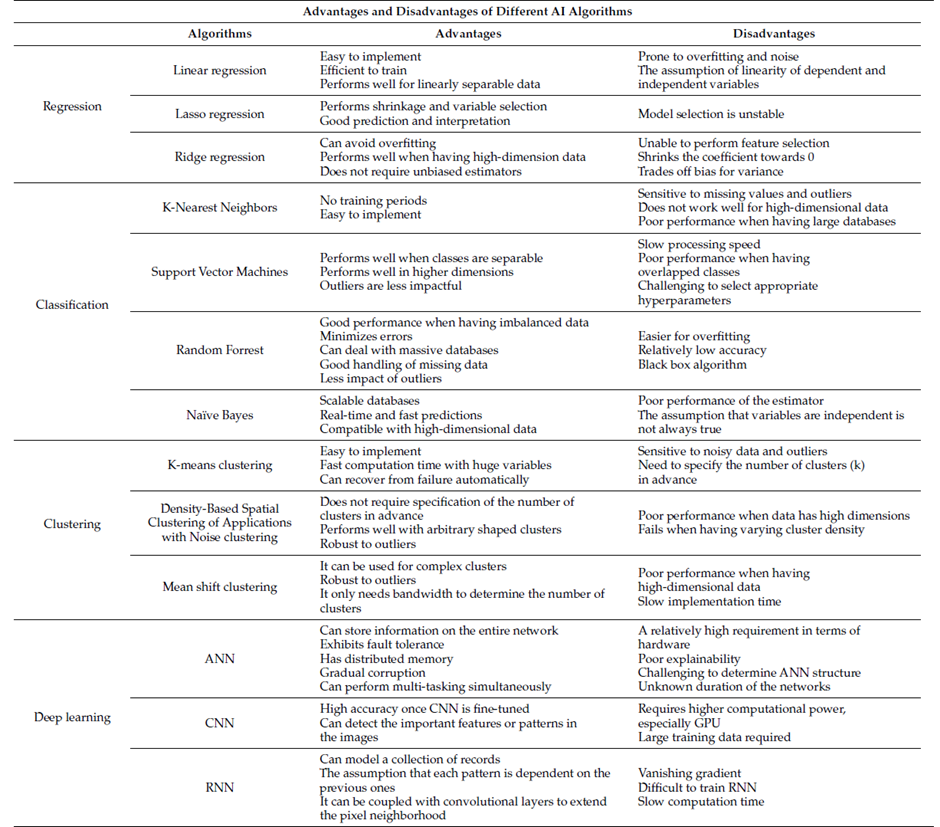
Predictive Performance Evaluation of the Model and Explainability
The step after the ML modelling process is the evaluation of the predictive performance of the models. There are several metrics that can be used, such as regression metrics and classification metrics. For the first one, usually the coefficient of determination (R2), the mean squared error (MSE), the root mean squared error (RMSE), and the mean absolute error (MAE) are most widely used. For classification modelling tasks, the starting point usually includes a matrix that calculates more than one metrics, such as accuracy precision, recall, F1-score, sensitivity, and specificity, followed by an additional evaluation metrics in case of an imbalanced dataset.
Once the model has been evaluated, other two steps need to be taken: these are the feature importance and the model explainability. Feature importance is indicated by the scores of all the variables that have been used in the model for the prediction. Therefore, a high score means that the specific variable has a significant effect on the model. The model explainability shows instead how trustworthy each prediction is. Nowadays, some sophisticated and advanced techniques can be applied to explain models.
Applications of AI in Solid Dosage Forms
Solid dosage forms include tablets, powders, and granules – the most common forms of drugs. Researchers started to use AI to investigate solid dosage forms in the 1990s. The interest spread quickly, with publications related to AI in solid dosage forms increasing by 100% each year since 2015. Importantly, different algorithms should be applied to different solid dosage formulations and for the specific application, as can be seen in Table 3.
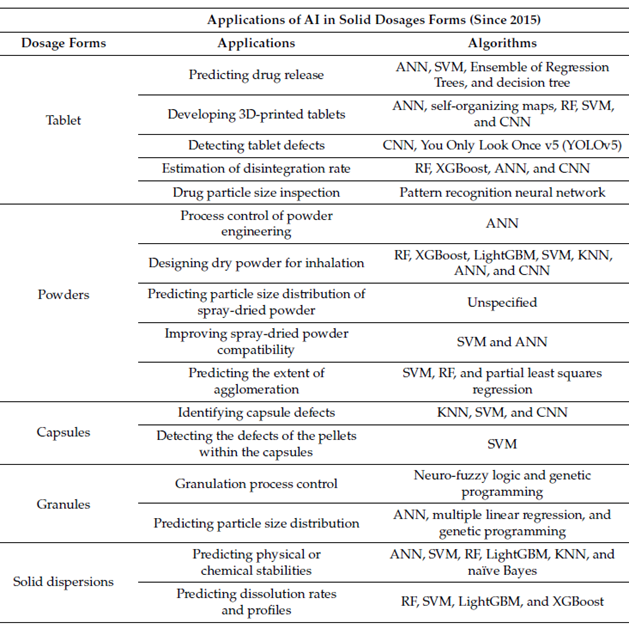
Tablets
Tablets are the most popular solid dosage form containing a mixture of APIs and excipients. During the product development process, a lot of parameters like pressure and tablet geometry, can influence the drug release profile. Additionally, in vitro studies require specific equipment, rendering the whole process time-consuming. AI can represent in that case a fundamental tool to assist scientists in predicting important details in the drug formulations, improving the product development process by saving time and costs.
When tablets are 3D printed, several methods involving a variety of parameters can be used. An additional aspect that can simplify the drug release profile is the use of AI technologies during the design process of a drug, allowing the reduction of experimental workload and the optimization of the 3D printing process.
An additional example of the importance of AI technologies is their application in tablet defects detection. Tablet defects are common during the manufacturing process, and they include cracking, capping, binding, and sticking. Over the years, the manual screen has been substituted by X-ray computer tomography (XRCT), which scientists have now combined with the deep learning technique, further optimizing the process of defects detection.
Powders
Powders are the oldest pharmaceutical dosage forms, and they consist of finely divided particles with sizes typically between 10 nm and 1000 µm. AI technologies have been applied in the process control during powder engineering, focussing particularly in obtaining the optimal particle sizes. The size of the particles is an important indicator that can influence the surface area, solubility, bioavailability of a drug. Recent studies applied AI in the process of controlling product quality and critical properties during the particle engineering process.
AI tools can also be valuable in predicting aerosol performance. Dry powders are widely used in inhaler devices to deliver the drug formulations into human lungs. The aerosol performance of dry powders is essential for their product development. AI can predict important parameters such as the fine particle fraction (FPF), or the median mass aerodynamic diameter (MMAD), contributing to their product development process.
Other Solid Dosage Forms
Other solid dosage forms are capsules, granules, and solid dispersions. Capsules are drugs that have been enclosed in a shell made from gelatin. AI methods applied to the development of capsule-based formulations are to date still limited in the literature. Similarly, some recent studies are starting to show the possible application of AI tools to the manufacturing process of granules, which consist of aggregates of powder particles with drug excipients, focusing on predicting their particle sizes. Finally, solid dispersions represent a solid dosage form that is strongly affected by environmental factors such as heat, moisture, and storage time, rendering this form physically and chemically unstable. AI techniques have been employed to predict characteristics like physical and chemical stability, alongside with dissolution rate and dissolution profiles.
Conclusions
This article highlights how AI technologies can help the development of solid dosage formulations and shows their potential to revolutionize the drug development pipeline. AI tools can predict several properties of solid dosage forms at low cost, providing fundamental guidance for scientists.
Next Steps
Learn more about the CSD.
Follow the link to the full article: Emerging Artificial Intelligence (AI) Technologies Used in the Development of Solid Dosage Forms. Pharmaceutics 2022, 14, 2257.
To discuss further and/or request a demo with one of our scientists, please contact us via this form or .